EdgeMine
Process mining on sensor data poses new challenges, such as the distributed and geographically spread nature of sensors nodes and their resource-constraints. This project, EdgeMine, will address these challenges and devise new approaches to (1) distributed, locality-aware process mining and (2) process mining on resource-constrained IoT devices. In the distributed mining setting of EdgeMine, each sensor node will locally compute a partial mining result, i.e., process fragments. Each fragment represents a partial data-flow graph of the process, which the sensor then forwards to neighboring sensors or edge devices, where it will be merged with process fragments of other sensors in a stepwise manner. With each step in this analysis chain, more fragments will be added in a distributed manner and the partial process graph will grow step by step to become a complete process model. Overall, the result will be a distributed data-flow graph, and we will map its computation onto physically distributed and heterogeneous IoT devices. Our results will push processing and analytics of data as close to the data sources as possible by distributing it over geographically scattered sensors, edge and cloud.
In this project, we will focus on the following research questions: (1) How can we devise locality-aware, distributed process-mining algorithms so that their data-flow aligns efficiently with the underlying topology of a physically distributed network of, for example, sensor nodes and without the need to collect data at a central location? (2) How can we devise resource-efficient process-mining algorithms that match the limited, often heterogeneous computing-resources in a network of IoT sensors, edge and cloud devices? (3) How can we automate the distribution and mapping of the algorithms devised in this project onto dynamic IoT networks and to dynamically adapt these to changing requirements, resources, and network topologies? (4) How can we devise benchmarks for systematic, fair and reproducible benchmarking of distributed, resource-efficient and adaptive process-mining algorithms, i.e., our research results?
The synergies of SOURCED, and especially the collaborations with experts in process mining, data mining, privacy, and software engineering will enable novel approaches. For example, distributed process mining, devised in EdgeMine, will serve as an enabler for scalable and privacy-aware process mining in SOURCED. Moreover, the explainability of process mining devised in SOURCED will guide us in this project to separate the important data from the unimportant data and thereby improve the resource-efficiency. Similarly, the works on uncertainty of data, will help EdgeMine to deal with noisy data. The Tiny House, which will be set up as part of SOURCED, will serve as a platform for benchmarking distributed process mining algorithms we devise in EdgeMine.
Since event logs often include sensitive personal information, the need for privacy awareness is urgent and imperative. In particular, distributed event sources are often recorded "close to the human" and offer even more intimate insights into a person's private life. Examples include tracking the locations of patients and doctors in hospitals or monitoring the tasks a worker performs. We will investigate distributed algorithms that push the process mining to the sources and thereby minimize and aggregate the data early in order to achieve provable privacy guarantees such as local differential privacy. To this end, we will collaborate closely with EdgeMine to investigate the challenges of distributed process Mining and AbstractMine to work on privacy-by-design principles for advanced notions of events, event transitions, and traces. By doing this, we will iteratively explore more complex scenarios and their boundaries.
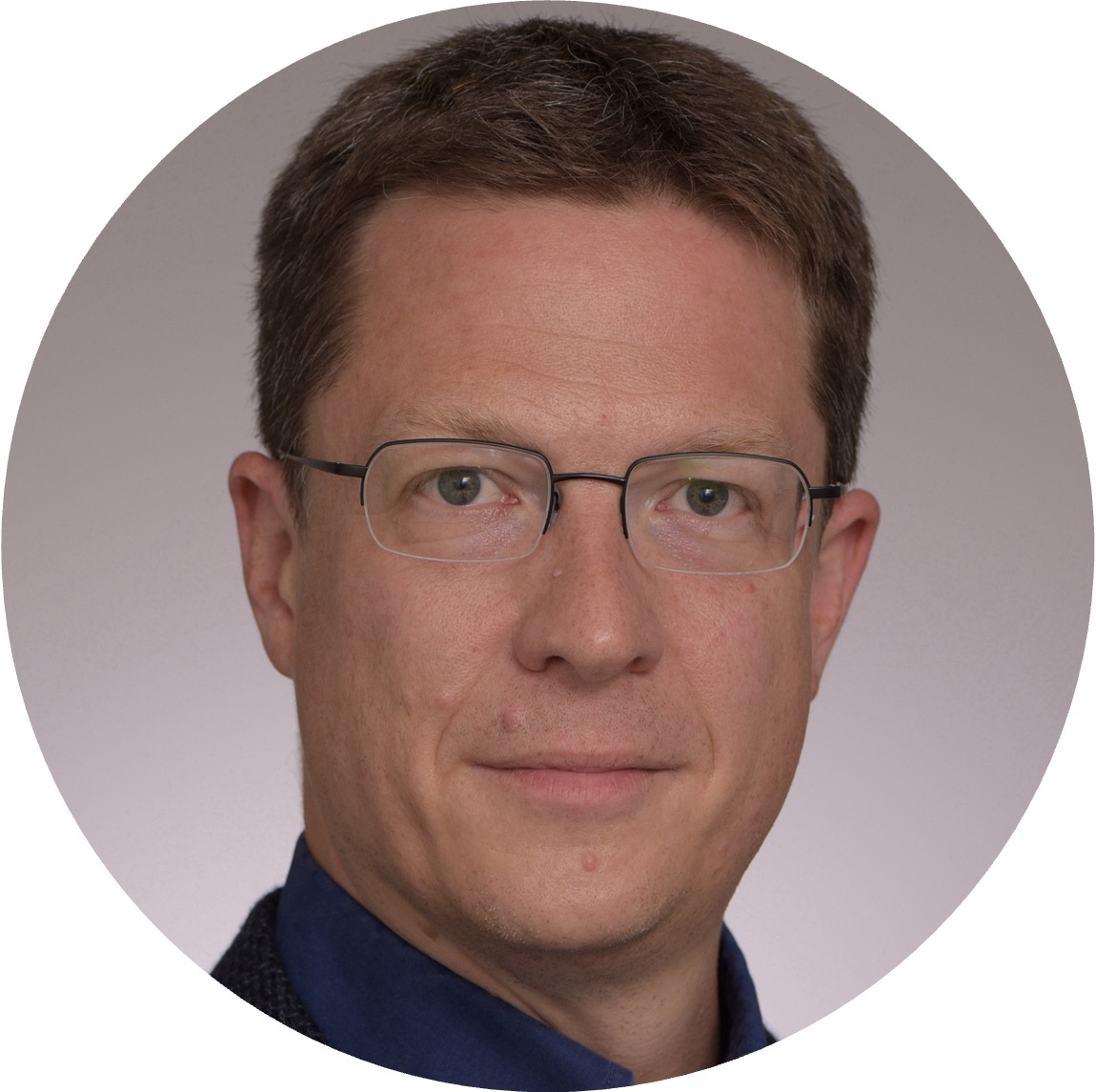
Prof. Dr. Olaf Landsiedel
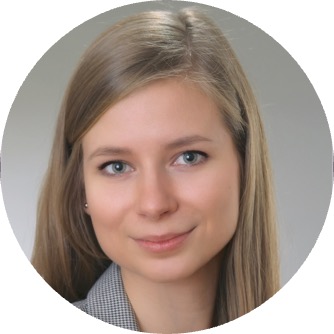
Julia Andersen