PrivateMine
Since event logs often include sensitive personal information, the need for privacy awareness is urgent and imperative. In particular, distributed event sources are often recorded "close to the human" and offer even more intimate insights into a person's private life. Examples include tracking the locations of patients and doctors in hospitals or monitoring the tasks a worker performs. We will investigate distributed algorithms that push the process mining to the sources and thereby minimize and aggregate the data early in order to achieve provable privacy guarantees such as local differential privacy. To this end, we will collaborate closely with EdgeMine to investigate the challenges of distributed process Mining and AbstractMine to work on privacy-by-design principles for advanced notions of events, event transitions, and traces. By doing this, we will iteratively explore more complex scenarios and their boundaries.
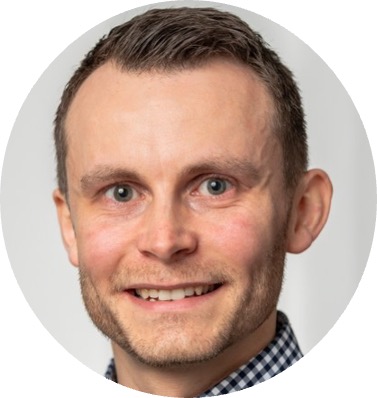
Prof. Dr. Florian Tschorsch
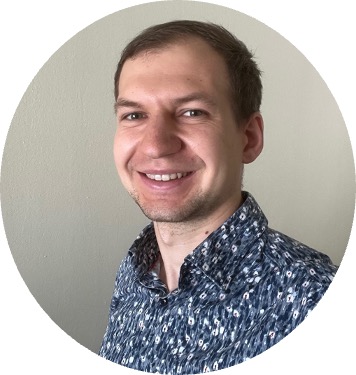
Maximilian Weisenseel